AlphaFold: A Game-Changer in Protein Structure Prediction, But Verification Remains Key
Google DeepMind's AlphaFold is a revolutionary AI algorithm predicting protein structures from their amino acid sequence. This article explores AlphaFold's potential to transform drug discovery, molecular medicine, and our understanding of life itself while highlighting the importance of experimental verification for accurate results.
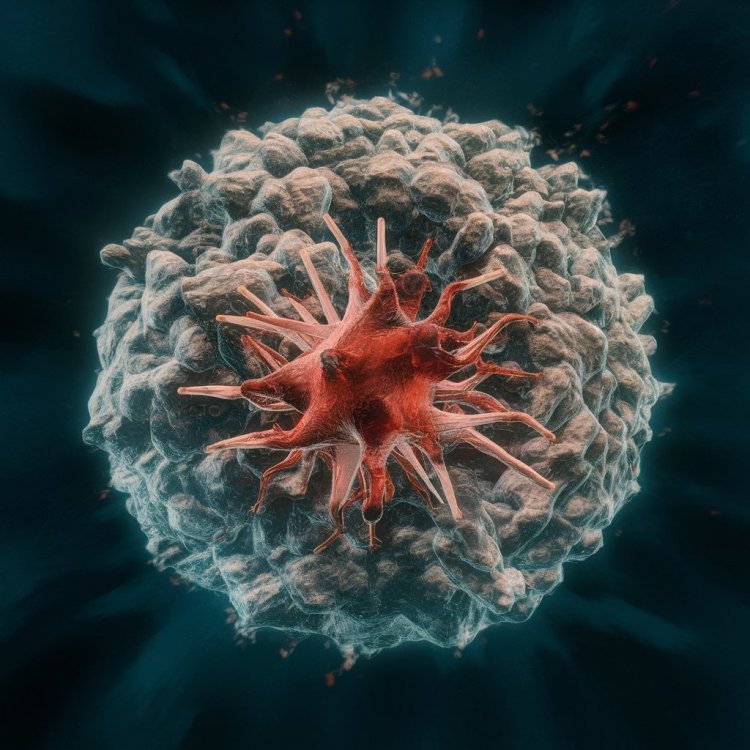
AlphaFold: A Game-Changer in Protein Structure Prediction, But Verification Remains Key
Proteins are the workhorses of our cells, playing critical roles in virtually every biological process. Understanding their intricate 3D structures is essential for unraveling the mysteries of life and developing new drugs. Traditionally, determining protein structures has been a time-consuming and expensive endeavor, often requiring complex experimental techniques like X-ray crystallography. However, Google DeepMind's groundbreaking algorithm, AlphaFold, has emerged as a revolutionary tool with the potential to transform protein science.
AlphaFold: Predicting Protein Structures with Unprecedented Accuracy
AlphaFold utilizes the power of deep learning, a form of artificial intelligence (AI), to predict protein structures from their amino acid sequence, the building blocks of proteins. By analyzing vast amounts of data on known protein structures, AlphaFold can identify patterns and relationships that allow it to accurately predict the 3D conformation of a new protein. This is a significant leap forward, offering researchers a faster, more cost-effective way to gain insights into protein function.
The latest iteration, AlphaFold 3, has further enhanced these capabilities. It not only predicts protein structures with remarkable accuracy but also delves into protein-protein and protein-ligand interactions. This ability to model how proteins interact with other molecules, including potential drugs, opens up exciting avenues for drug discovery.
Revolutionizing Drug Discovery with AlphaFold
Traditionally, drug discovery involves a lengthy and expensive process of identifying molecules that can bind to a specific protein target and disrupt its disease-causing function. AlphaFold's ability to predict protein structures and interactions can significantly accelerate this process. By providing researchers with a clear picture of the target protein's 3D structure, AlphaFold allows for the virtual screening of millions of potential drug candidates. This can lead to the rapid identification of promising leads with a higher chance of success in clinical trials.
Beyond Drug Discovery: The Wider Impact of AlphaFold
The implications of AlphaFold extend far beyond drug discovery. With its ability to predict protein structures across the vast spectrum of life, AlphaFold can revolutionize various fields within biology and medicine. Here are some exciting possibilities:
- Understanding Disease Mechanisms: By elucidating the structures of disease-associated proteins, AlphaFold can shed light on the underlying mechanisms of various diseases, paving the way for the development of more targeted therapies.
- Development of Biomaterials: The ability to predict protein structures can aid in the design of novel biomaterials with specific properties for tissue engineering and regenerative medicine.
- Enhancing Enzyme Design: AlphaFold can be instrumental in engineering enzymes with improved efficiency and selectivity for various industrial applications.
Challenges and the Importance of Experimental Verification
While AlphaFold represents a significant breakthrough, it's crucial to recognize that it's not a perfect solution. Despite its impressive accuracy, AlphaFold's predictions still require experimental verification. Here are some key challenges to consider:
- Limited Training Data: AlphaFold's predictions rely heavily on the quality and quantity of data used to train the model. As the protein universe is vast and constantly expanding, there will always be proteins with unique structures that fall outside the scope of existing data.
- Conformational Flexibility: Proteins are not static structures; they can adopt various conformations depending on their environment and interactions with other molecules. AlphaFold may not always capture the full range of conformational flexibility.
- Integration with Biological Systems: Predicting protein structure is only one piece of the puzzle. Understanding how proteins function within the complex biological milieu requires integrating structural information with other biological data.
Conclusion: AlphaFold – A Powerful Tool with a Promising Future
AlphaFold represents a paradigm shift in protein structure prediction, offering unprecedented speed and accuracy. Its impact on various fields in biology, medicine, and beyond has the potential to be transformative. However, it's important to remember that AlphaFold is a tool, not a replacement for experimental methods. Integrating AlphaFold predictions with traditional techniques and ongoing research efforts will be crucial to fully unlock its potential and revolutionize our understanding of the protein universe.
What's Your Reaction?
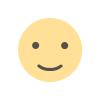



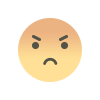
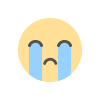
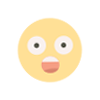